Artificial Intelligence Research
Artificial Intelligence research at UR includes work on knowledge representation and reasoning, machine learning, dialog systems, statistical natural language processing, automated planning, AI-based assistive technology, and computer vision. We collaborate with the departments of linguistics and brain and cognitive sciences. We participate in the Center for Language Sciences, the Center for Visual Science, and the Goergen Institute for Data Science and Artificial Intelligence.
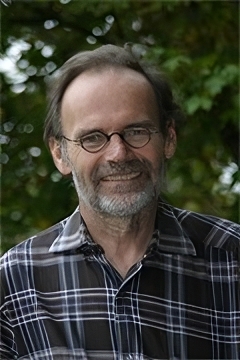
Allen, James F.
Professor Emeritus of Computer Science
- Web Address
- Website
Interests: Natural language understanding; Discourse; Knowledge representation; Common-sense reasoning; Planning
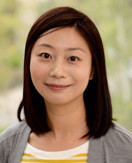
Bai, Zhen
Biggar Family Fellow in Data Science, Goergen Institute for Data Science and Artificial Intelligence
Assistant Professor of Computer Science
- Office Location
- 3007 Wegmans Hall
- Telephone
- (585) 275-7758
- Web Address
- Website
Interests: Human-Computer Interaction; Augmented Reality; Tangible User Interfaces; Embodied Conversational Agent; Technology-Enhanced Learning; Computer-Supported Collaborative Work; Assistive Technology; Machine Learning for HCI
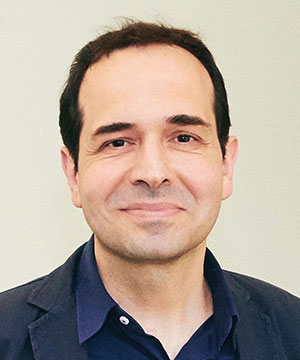
Cetin, Mujdat
Robin and Tim Wentworth Director, Goergen Institute for Data Science and Artificial Intelligence
Professor of Electrical and Computer Engineering
Professor of Computer Science
Director, New York State Center of Excellence in Data Science
- Office Location
- 1211 Wegmans Hall and 719 Computer Studies Building
- Telephone
- (585) 276-5061
Interests: computational sensing and imaging as applied to radar and biomedical imaging; probabilistic methods for image and video analysis as applied to biomedical image analysis, microscopic neuroimaging, and computer vision; signal processing and machine learning for brain-computer/machine interfaces, with applications for alternative communication and rehabilitation for patients and monitoring of cognitive states
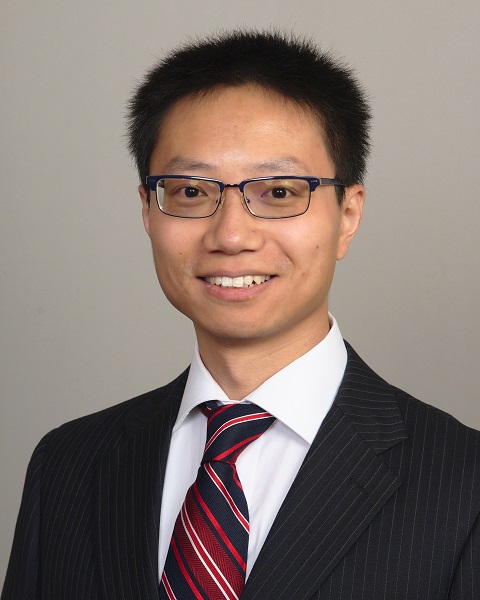
Duan, Zhiyao
Associate Professor of Electrical and Computer Engineering and of Computer Science
- Office Location
- 720 Computer Studies Building
- Telephone
- (585) 275-5302
- Web Address
- Website
Interests: Computer Audition; Audio Signal Processing; Machine Learning; Music Information Retrieval
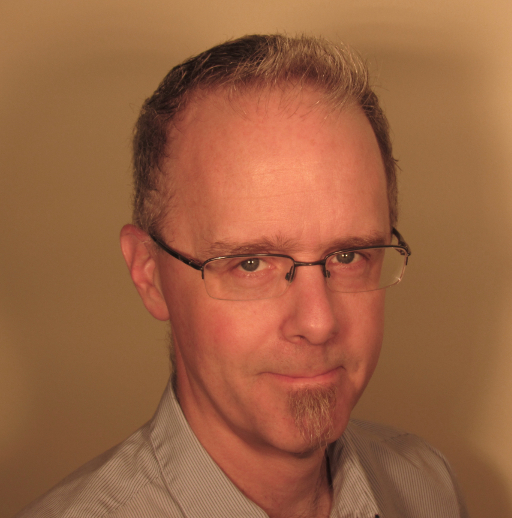
Ferguson, George
Professor of Computer Science (Instruction) Co-Director Computer Science Undergraduate Program
- Office Location
- 2103 Wegmans Hall
- Web Address
- Website
Interests: Artificial intelligence; Intelligent agents; Agent communication languages; Agent architectures; User interfaces: speech recognition, natural language understanding, dialogue, conversational agents; Temporal reasoning: representation, planning, scheduling; Semantic web: ontologies, knowledge-based systems
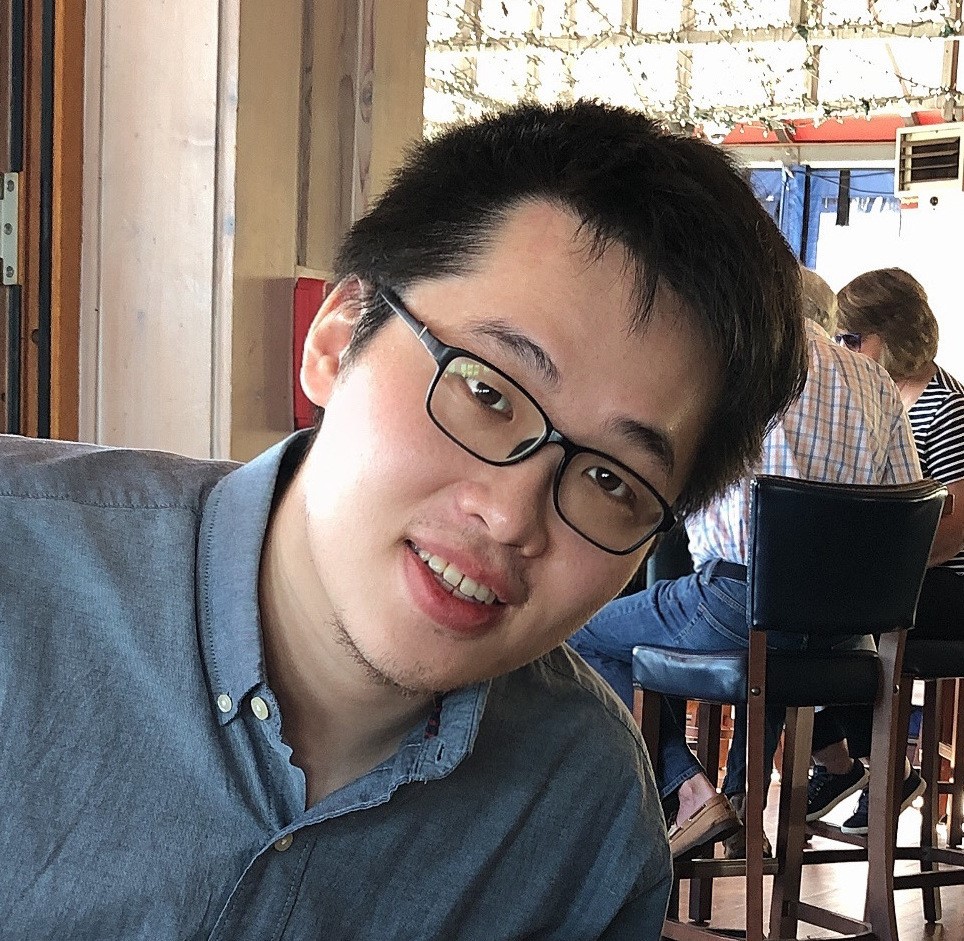
Geng, Tong (Tony)
Assistant Professor of Electrical and Computer Engineering and Computer Science
- Office Location
- 723 Computer Studies Building
- Telephone
- (585) 275-1735
- Web Address
- Website
Interests: Computer architecture; Graph learning; Machine learning; Accelerators for AI; High-performance computing
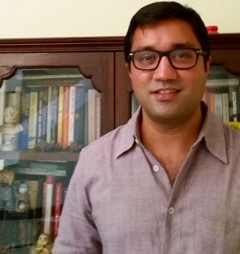
Ghoshal, Gourab
Professor of Physics
Professor of Computer Science
- Office Location
- 464 Bausch & Lomb Hall
- Telephone
- (585) 276-7748
- Web Address
- Website
Interests: theoretical condensed-matter physics and data science
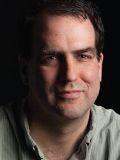
Gildea, Daniel
Professor of Computer Science
- Office Location
- 3019 Wegmans Hall
- Telephone
- (585) 275-7230
- Web Address
- Website
Interests: Machine translation; Semantic parsing; Algorithms for natural language processing
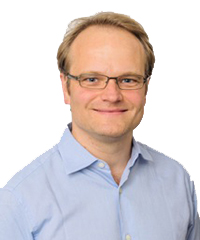
Haefner, Ralf
Associate Professor of Brain & Cognitive Sciences
Associate Professor of Physics
Associate Professor of Computer Science
- Office Location
- 318 Meliora Hall
- Web Address
- Website
Interests: Computational Neuroscience; Perceptual decision-making; Probabilistic inference; Selective attention; Neural population encoding and decoding; Depth perception/processing of binocular disparity
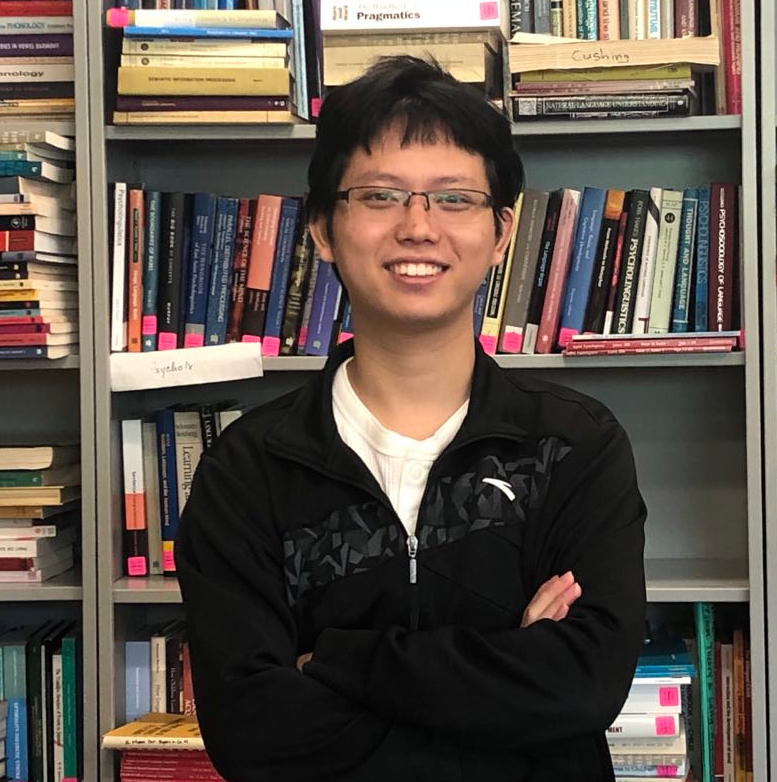
He, Hangfeng
Assistant Professor of Computer Science and Data Science
- Office Location
- 3009 Wegmans Hall
- Telephone
- (585) 275-8848
- Web Address
- Website
Interests: Machine learning; Natural language processing; Reasoning
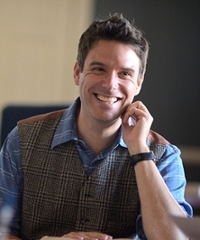
Heyworth, Gregory
Professor of English
Professor of Computer Science
Professor of History
Director, Lazarus Project
- Office Location
- 408 Morey Hall
- Telephone
- (585) 275-2548
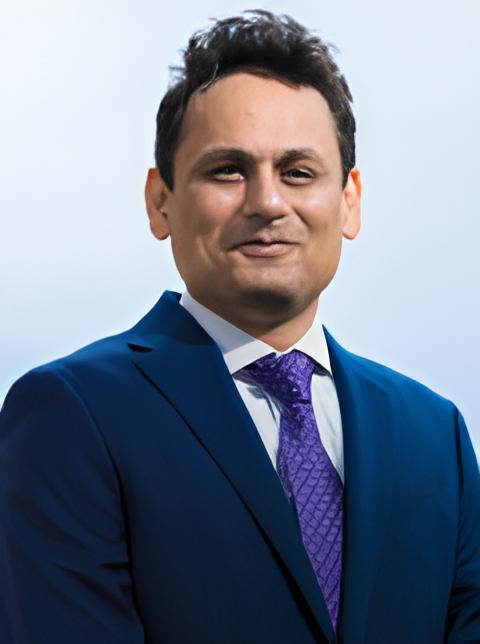
Hoque, Ehsan
Professor of Computer Science
- Office Location
- 3013 Wegmans Hall
- Telephone
- (585) 275-1351
- Web Address
- Website
Interests: Human Computer Interaction; Artificial Intelligence ; Interactive machine learning; Health and wellbeing; Future of skills
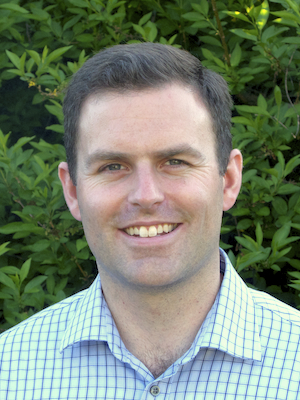
Howard, Thomas M.
Associate Professor of Electrical and Computer Engineering
Associate Professor of Computer Science
- Office Location
- 732 Computer Studies Building
- Telephone
- (585) 275--375
Interests: Artificial Intelligence; Robotics; Motion Planning; Human-Robot Interaction
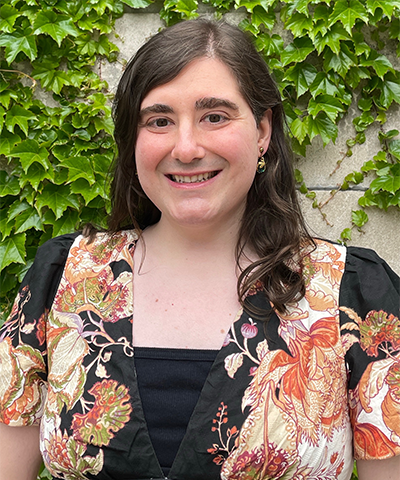
Iordan, Coraline Rinn
she/her/hers
Assistant Professor, Brain and Cognitive Sciences, Neuroscience
- Office Location
- 308 Meliora Hall
- cora@rochester.edu
- Web Address
- Website
Interests: visual and semantic cognition; learning; attention; neuroplasticity; real-time fMRI; neurofeedback; machine learning; neural networks; data science
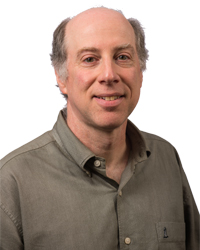
Jacobs, Robert A.
Professor, Brain and Cognitive Sciences, Computer Science, and the Center for Visual Science
- Office Location
- 306 Meliora Hall
- Telephone
- (585) 275-0753
- Web Address
- Website
Interests: cognitive and perceptual learning; neural computation
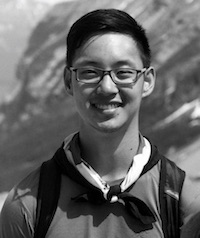
Kahng, Anson
Assistant Professor of Computer Science and Data Science
- Office Location
- 2401 Wegmans Hall
- Web Address
- Website
Interests: Computational social choice; Democracy and computer science; Economics and computation; Artificial intelligence; Theoretical computer science; Algorithmic game theory
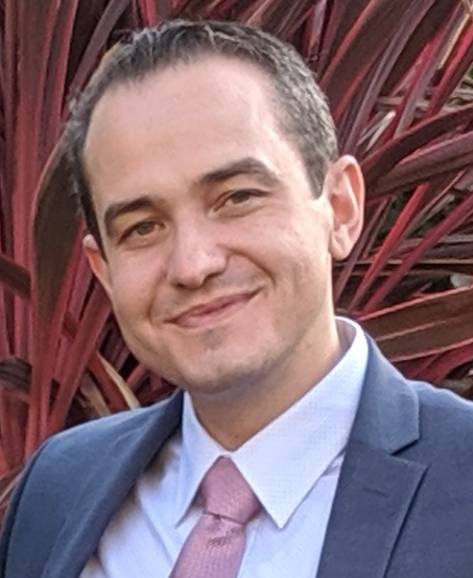
Kanan, Christopher
Associate Professor of Computer Science
- Office Location
- 3017 Wegmans Hall
- Web Address
- Website
Interests: Artificial Intelligence; Deep Learning; Computer Vision; Cognitive Science; Applied Machine Learning (e.g. Medical Computer Vision)
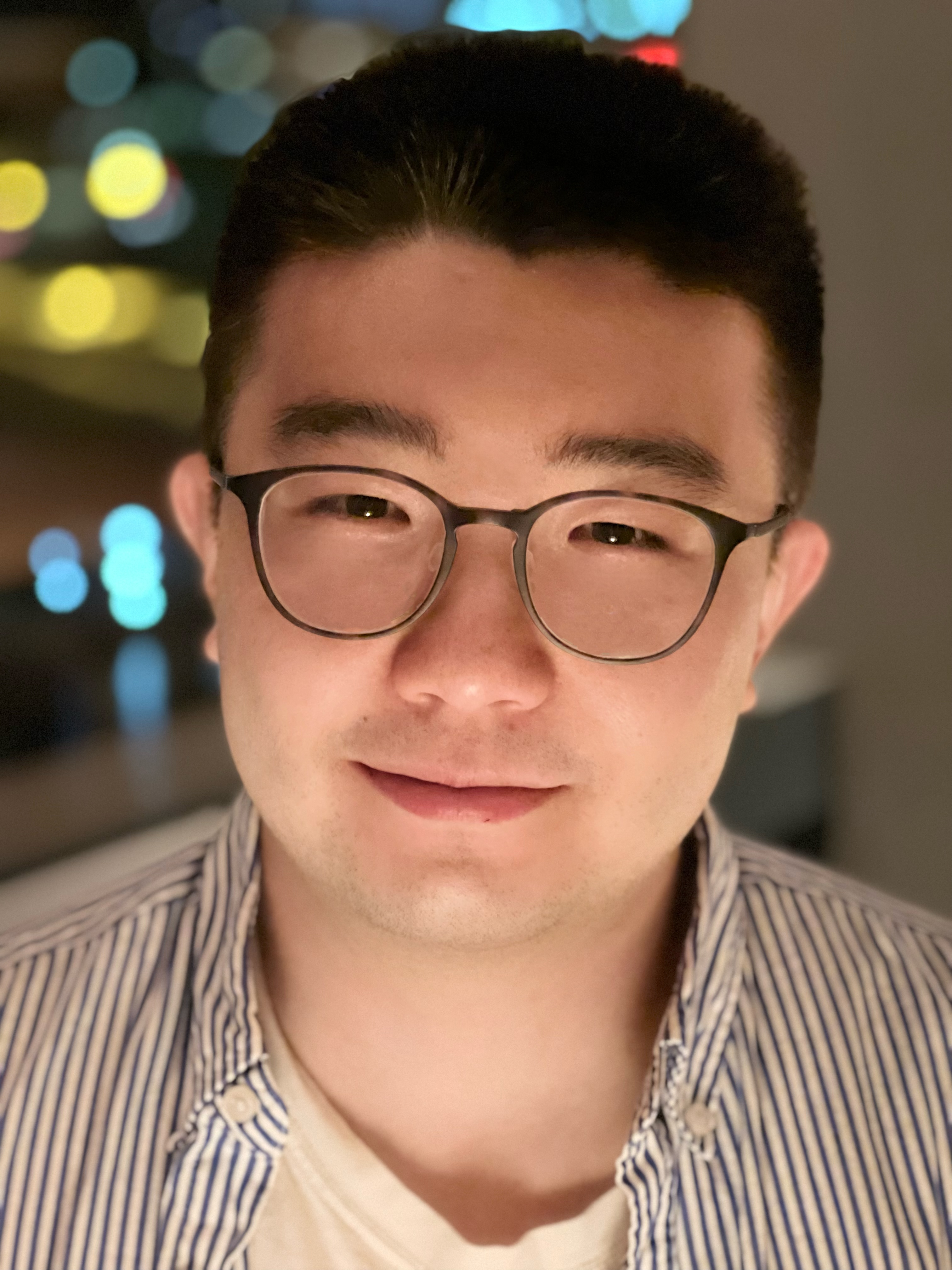
Kang, Jian
Assistant Professor of Computer Science
- Office Location
- 3011 Wegmans Hall
- Web Address
- Website
Interests: Data mining; Computational social science; Uncertainty quantification; Algorithmic fairness; Trustworthy AI; Machine learning
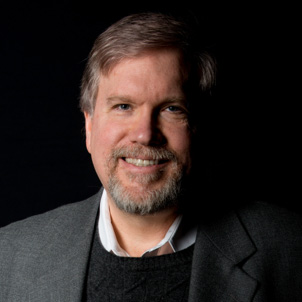
Kautz, Henry
Professor Emeritus of Computer Science
- Web Address
- Website
Interests: Artificial intelligence; Pervasive computing; Data science
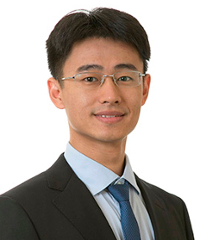
Liang, Jiaming
Assistant Professor of Computer Science and Data Science
- Office Location
- 2403 Wegmans Hall
- Web Address
- Website
Interests: Mathematical and numerical optimization; Sampling algorithms; Stochastic programming; Algorithmic game theory
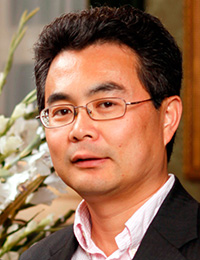
Luo, Jiebo
Albert Arendt Hopeman Professor of Engineering
Professor of Computer Science
- Office Location
- 3101 Wegmans Hall
- Telephone
- (585) 276-5784
- Web Address
- Website
Interests: Computer Vision; Machine learning; Data Mining; Social Media; Biomedical Informatics; Human Computer Interaction; Ubiquitous and Mobile Computing
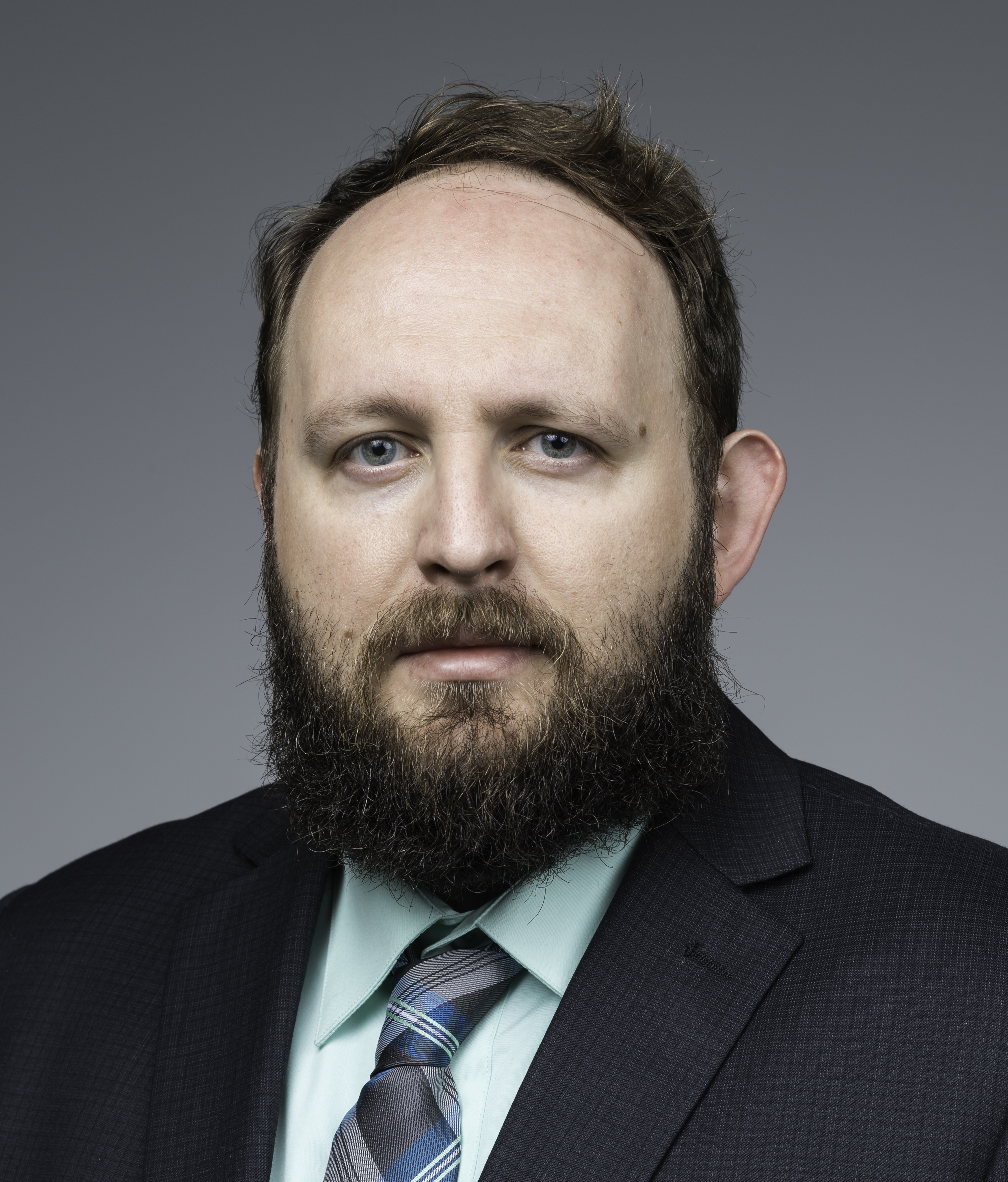
Purtee, Adam
Associate Professor of Computer Science (Instruction)
- Office Location
- 2109 Wegmans Hall
- Web Address
- Website
Interests: Natural language understanding ; Knowledge representation ; Commonsense reasoning ; Machine Learning ; Numerical programming
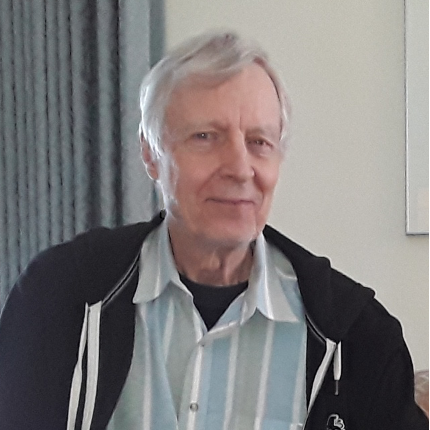
Schubert, Lenhart K.
Professor Emeritus of Computer Science
- Web Address
- Website
Interests: Language and dialogue ; Knowledge representation and inference; Schema learning and schema-based behavior ; Self-motivated agents
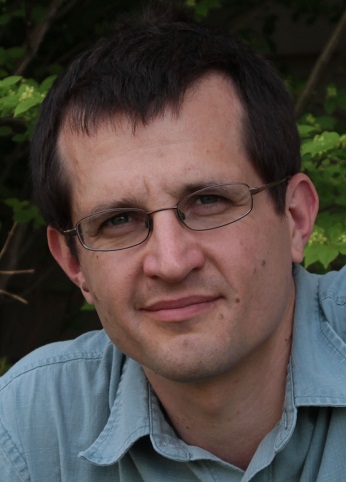
Stefankovic, Daniel
Professor of Computer Science
- Office Location
- 2315 Wegmans Hall
- Web Address
- Website
Interests: Theoretical computer science: graph theory, combinatorics, Fourier transform, Markov chains/counting, learning theory, phylogeny, game theory, graph equations, routing
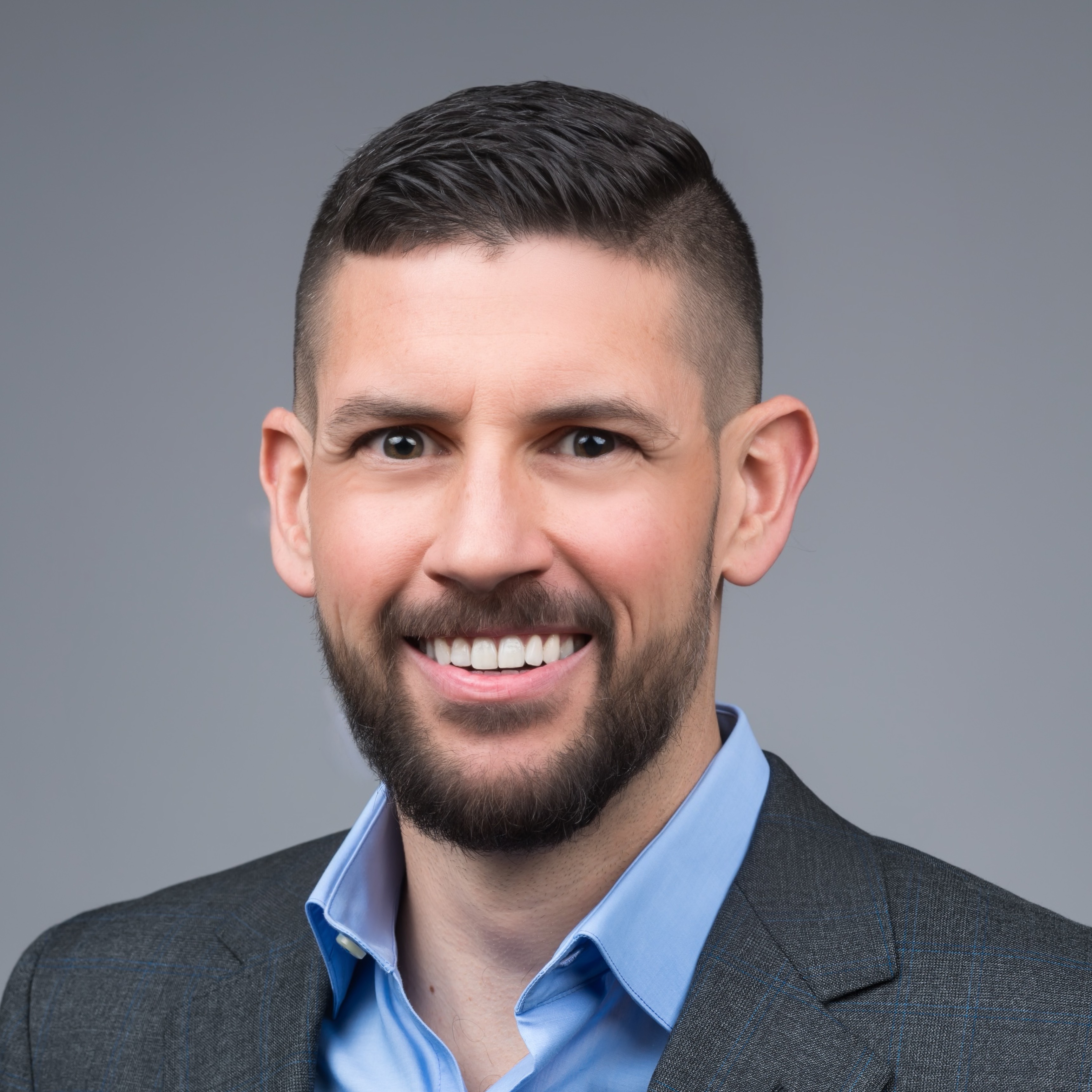
White, Aaron
Associate Professor of Linguistics
Associate Professor of Computer Science
Director of FACTS.lab
- Office Location
- 511A Lattimore Hall
- Web Address
- Website
Interests: Computational Linguistics; Natural Language Semantics
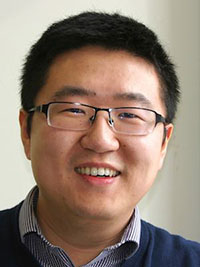
Xu, Chenliang
Associate Professor of Computer Science
- Office Location
- 3005 Wegmans Hall
- Web Address
- Website
Interests: Computer Vision; Video Analysis; Machine Learning; Deep Learning; Multimodal Modeling